Unlocking the Future: Labeling Tools for Machine Learning
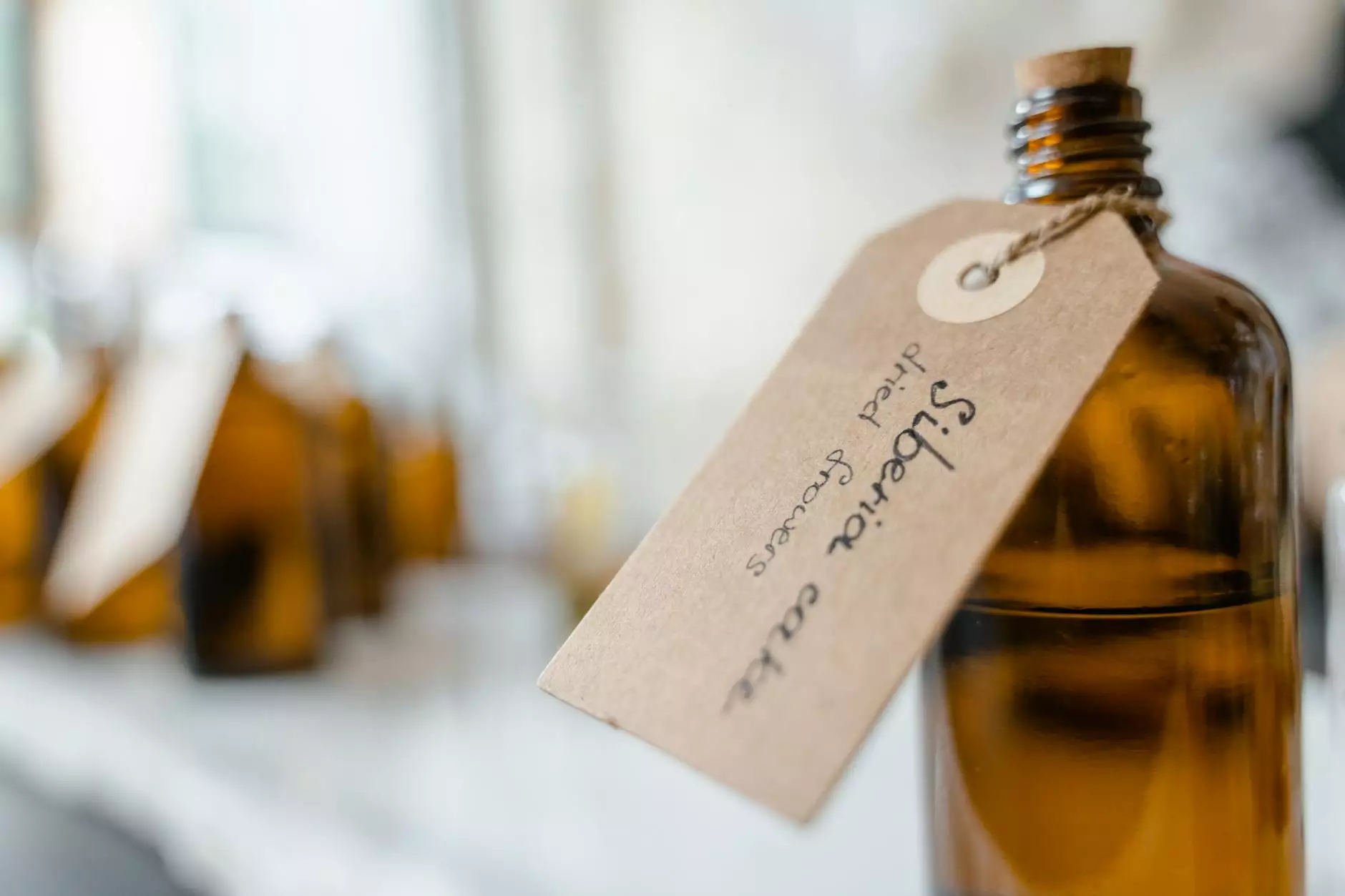
The rise of artificial intelligence and machine learning has revolutionized various industries, from healthcare to finance to e-commerce. However, the success of machine learning models heavily relies on the quality of the data they are trained on. This is where labeling tools for machine learning come into play. In this comprehensive article, we will explore the significance of these tools, the different types available, and how businesses can leverage them to gain a competitive edge.
Understanding Data Annotation
At the core of machine learning is a process called data annotation. This involves the meticulous task of labeling data accurately. Labeling tools enable organizations to tag and classify data, providing the necessary context for machine learning algorithms to learn from. Without proper labeling, machine learning models struggle to identify patterns, leading to subpar performance.
The Importance of Labeling Tools
Labeling tools are essential for several reasons:
- Data Quality: High-quality labeled data directly impacts the accuracy of machine learning models.
- Efficiency: Automated labeling tools save time and reduce human error in the annotation process.
- Scalability: As businesses grow, so does the volume of data. Labeling tools can scale to meet increasing demands.
- Collaboration: Many tools offer features that allow teams to collaborate seamlessly on data annotation projects.
Different Types of Labeling Tools for Machine Learning
When it comes to labeling tools for machine learning, there are several types that cater to different needs and data types:
1. Image Annotation Tools
Image annotation tools allow users to label images by drawing bounding boxes, polygons, or segmenting objects within the image. These tools are vital for training models in fields such as computer vision and autonomous vehicles.
2. Video Annotation Tools
Video annotation tools extend image annotation capabilities to the temporal dimension. They allow for the labeling of frames in videos, which is crucial for training models in areas like action recognition and surveillance.
3. Text Annotation Tools
Text annotation tools are used to label textual data, which can include sentiment analysis, named entity recognition, and classification tasks. These tools simplify the process of extracting valuable insights from unstructured data.
4. Audio Annotation Tools
Audio annotation tools facilitate the labeling of audio segments, identifying different sounds or transcribing speech. These tools are essential for training models in areas like speech recognition and music analysis.
KeyLabs.ai: A Leader in Data Annotation Tools
At the forefront of this innovative space is KeyLabs.ai. Specializing in data annotation tools and data annotation platforms, KeyLabs.ai offers robust solutions that simplify the labeling process. Here’s why businesses should consider their tools:
Advanced Technology
KeyLabs.ai employs cutting-edge technology in their labeling tools, including machine learning algorithms that enhance the accuracy of annotated data. Their platform is designed to adapt to various data types, making it versatile for different industries.
User-Friendly Interface
The tools provided by KeyLabs.ai feature an intuitive user interface that allows teams to start annotating data quickly and efficiently. This ease of use is crucial for ensuring that even less technical staff can contribute to the labeling process.
Customizable Solutions
Every business has unique needs, and KeyLabs.ai recognizes this by offering customizable solutions. Companies can tailor the labeling workflows to fit their specific requirements, optimizing productivity and outcomes.
Collaboration Features
With built-in collaboration features, teams can work together in real time on annotation projects, ensuring consistency and accuracy across the board. This fosters a more dynamic working environment and speeds up the labeling process.
How to Choose the Right Labeling Tools for Machine Learning
Selecting the appropriate labeling tool is critical for the success of a machine learning project. Here are some factors to consider:
1. Data Type
Identify the type of data you will be working with (images, text, audio, etc.) and choose a tool that specializes in that area.
2. Scalability
Consider the future needs of your organization. Opt for tools that can scale up as your data volume increases.
3. Integration Capabilities
Choose labeling tools that integrate seamlessly with your existing workflows and software to avoid compatibility issues.
4. Budget
Evaluate your budget and find a solution that offers the best value for your investment while meeting your labeling needs.
The Future of Labeling Tools in Machine Learning
The future of labeling tools is promising, with advances in artificial intelligence and machine learning driving innovation. Here are some trends to watch:
1. Increased Automation
As technology improves, we can expect to see more automated labeling tools that can handle complex tasks with minimal human intervention, further increasing efficiency.
2. Enhanced AI Integration
Future labeling tools will likely incorporate advanced AI capabilities that will assist in annotation, reducing the time needed for manual input and improving the accuracy of labels.
3. Focus on Quality Assurance
With the growing importance of data integrity, future tools will place a larger emphasis on quality assurance mechanisms to ensure that labeled data meets high standards.
Conclusion: The Crucial Role of Labeling Tools for Machine Learning
In a world driven by data, the importance of effective labeling tools for machine learning cannot be understated. KeyLabs.ai is paving the way with its innovative data annotation solutions that empower businesses to harness their data effectively. By investing in quality labeling tools, companies not only enhance the performance of their machine learning models but also secure a competitive advantage in their respective fields.
As businesses continue to embrace AI and machine learning, understanding and utilizing the best labeling tools for machine learning will be pivotal in unlocking new opportunities and driving innovation.